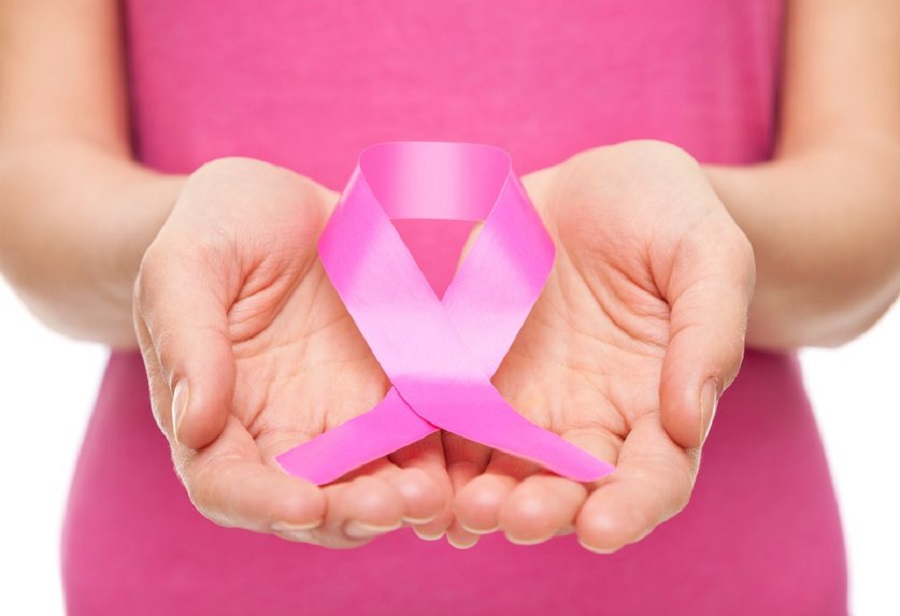
KOCHI:
The Artificial Intelligence & Computer Vision Lab of the Department of Computer Science, Cochin University of Science and Technology developed an ingenious technology for the grading of breast cancer in association with the Intel Technology Pvt. Ltd. Early detection and diagnosis of breast cancer will increase the chance of recovery and chance of survival. The non-invasive radiological diagnosis using ultrasound, mammography, and MRI are widely used for the diagnosis of breast cancer at present. In biopsy analysis, the breast cancer grading forms a significant parameter for prescribing patient specific treatment plans and for the disease prognosis due to its influential association with the survival chances of the patient.
Breast cancer grade is presently determined manually, by the microscopic investigation and analysis of the biopsy samples. The manual microscopic examination and grading is tedious, time-consuming, and expensive. It demands the automation of the process. A novel automated technique based on semi-supervised learning framework combined with the deep neural network based generative adversarial model, known as NASSGAN was developed by CUSAT
scientists for discriminating the different cancer grades. The proposed work has been published in the prestigious International Journal named IEEE Journal of Biomedical and Health Informatics.
Automation of cancer grading requires developing accurate and robust classifier models, that needs to be trained with a large amount of annotated data. The advancements in the field of digital pathology have been accelerated by the advent of whole slide imaging (WSI) techniques that can produce high resolution digital slides within a fraction of minutes at reasonable cost. These images are unlabeled and needs annotation to be done manually by proficient
pathologists.
It requires several such experts to annotate the same image to assure the fidelity of diagnosis which makes annotation much expensive, especially for procuring a decent amount of labelled images. The proposed model developed based on semi supervised learning (SSL) can considerably reduce the need of annotated data, reduce the time and cost. Intel has developed a hardware architecture with Intel Xeon scalable processors using distributed training to reduce the training time and to support the experimentation with multiple image resolutions without memory constraints. Four Intel Xeon scalable processors are used as a compute cluster without any deep learning accelerators for the model training.
The work was carried out under the expertise of Dr. Madhu S. Nair, Asso. Professor, Artificial Intelligence & Computer Vision Lab, Department of Computer Science, CUSAT and Dr. Asha Das, Former Research Scholar, Department of Computer Science, CUSAT and presently Assistant Professor, Department of Computer Science, Union Christian College, Aluva with the technical support of Vinod Kumar Devarampati, Intel Technology Asia Pte
Ltd, Singapore. Intel’s Engineering team of Vivek Kumar Rai and Rahul Tallamraju has also been a part of the work.